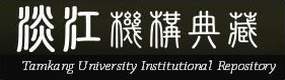 |
English
|
正體中文
|
简体中文
|
Items with full text/Total items : 64191/96979 (66%)
Visitors : 8389312
Online Users : 7828
|
|
|
Loading...
|
Please use this identifier to cite or link to this item:
https://tkuir.lib.tku.edu.tw/dspace/handle/987654321/98490
|
Title: | A Real-time Sign Language Recognition System for Hearing and Speaking Challengers |
Authors: | Hsieh, Chieh-Fu;Chen, Li-Ming;Huang, Ku-Chen;Hsieh, Ching-Tang;Yih, Chi-Hsiao |
Contributors: | 淡江大學電機工程學系;淡江大學體育事務處體 |
Keywords: | Sign language;Kinect;Human Machine Interface (HMI);hidden Markov model (HMM) |
Date: | 2014-07-12 |
Issue Date: | 2014-08-07 18:08:09 (UTC+8) |
Publisher: | Taipei: Asia-Pacific Education & Research Association |
Abstract: | Sign language is the primary means of communication between deaf people and hearing/speaking challengers. There are many varieties of sign language in different challenger community, just like an ethnic community within society. Unfortunately, few people have knowledge of sign language in our daily life. In general, interpreters can help us to communicate with these challengers, but they only can be found in Government Agencies, Hospital, and etc. Moreover, it is expensive to employ interpreter on personal behalf and inconvenient when privacy is required. It is very important to develop a robust Human Machine Interface (HMI) system that can support challengers to enter our society. A novel sign language recognition system is proposed. This system is composed of three parts. First, initial coordinate locations of hands are obtained by using joint skeleton information of Kinect. Next, we extract features from joints of hands that have depth information and translate handshapes. Then we train Hidden Markov Model-based Threshold Model by three feature sets. Finally, we use Hidden Markov Model-based Threshold Model to segment and recognize sign language. Experimental results show, average recognition rate for signer-dependent and signer-independent are 95% and 92%, respectively. We also find that feature sets including handshape can achieve better recognition result. |
Relation: | Proceedings of International Research Conference on Information Technology and Computer Sciences, pp.24-31 |
Appears in Collections: | [體育事務處] 會議論文 [電機工程學系暨研究所] 會議論文
|
Files in This Item:
File |
Description |
Size | Format | |
IRCITCS-266_A Real-time Sign Language Recognition System for Hearing and Speaking Challengers.pdf | 會議論文內容 | 613Kb | Adobe PDF | 955 | View/Open |
|
All items in 機構典藏 are protected by copyright, with all rights reserved.
|