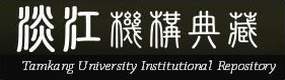 |
English
|
正體中文
|
简体中文
|
Items with full text/Total items : 64191/96979 (66%)
Visitors : 8386846
Online Users : 8481
|
|
|
Loading...
|
Please use this identifier to cite or link to this item:
https://tkuir.lib.tku.edu.tw/dspace/handle/987654321/94567
|
Title: | 整合聚類分析與類神經網路於區域淹水預測之研究 |
Other Titles: | Study of building regional flood inundation forecast models by integrating clustering analysis and artificial neural networks |
Authors: | 沈宏榆;Shen, Hung-Yu |
Contributors: | 淡江大學水資源及環境工程學系博士班 張麗秋;Chang, Li-Chiu |
Keywords: | 類神經網路;自身回饋機制的含外變數非線性自迴歸模式;自組特徵映射網路;淹水潛勢圖;未設測站淹水預測;區域淹水預測;artificial neural networks;Recurrent configuration of nonlinear autoregressive with exogenous inputs(R-NARX);self-organizing map(SOM);Potential inundation maps;Ungauged regional flood inundation forecasting model |
Date: | 2013 |
Issue Date: | 2014-01-23 14:46:43 (UTC+8) |
Abstract: | 近幾年世界各地水文極端事件明顯增多且強度增加,所造成的災害程度皆較以往劇烈,如2005年卡崔娜颶風、2009年莫拉克颱風、2010年泰國降下長達4個月的暴雨、2010年梅姬颱風與2011年颱風和暴風雨襲擊巴西,其所挾帶的豪大雨量與高降雨強度均帶來區域性的嚴重淹水災情,因此,推估淹水深與淹水範圍,有助於提供災情資訊,以減輕災害風險與損失。 傳統淹水模式需要大量的數值迭代計算時間,以獲得高解析度之淹水圖;而為了對各種不同的淹水狀況,實施適當的洪災防救的策略,需配合各種不同降雨情況之淹水圖與防災方案;因此,傳統二維淹水模擬模式需耗費較長的演算時間,對於洪災防救的緊急應變時間不足,使得無法提供即時淹水預警。為了建置即時淹水預警系統,本研究提出兩種區域淹水預測模式:CHIM(以聚類分析為基礎之複合型淹水模式與SOM-R-NARX(以自組特徵映射網路結合R-NARX之區域淹水模式)。 CHIM模式有資料前處理與模式建置兩階段步驟;在資料前處理階段,以K-Means聚類法將研究區域依淹水特性進行分類,以獲得各淹水分群之控制點;在模式建置階段則以R-NARX(自身回饋機制的含外變數非線性自迴歸模式),建立各淹水分群之控制點及多點淹水預測模式。SOM-R-NARX則以自組特徵映射網路(SOM)結合R-NARX之區域淹水模式,本模式由兩種模式結合預測區域淹水深與淹水範圍;SOM模式將研究區域之不同淹水圖進行分類,產生區域淹水拓樸圖,以獲得區域淹水分布之拓樸關係;R-NARX模式則進行預測研究區域之總淹水體積,將其總淹水體積預測值與SOM淹水拓樸圖中各神經元淹水總體積進行比較,找出總淹水體積最接近之神經元,並校正該神經元之權重(淹水深),以獲得區域淹水圖。 兩模式主要差異為CHIM先依淹水地點之淹水特性進行分類,再分別建置各分群之單點與多點淹水預測模式,控制點預測淹水深值提供局部區域淹水重要淹水資訊,以提升模式效率及精確度;而SOM-R-NARX則探討不同雨型與區域淹水空間分布之特性。本研究以宜蘭縣作為研究區域,結果顯示兩模式皆能大幅提昇淹水預測的時效性及可行性,而其中SOM-R-NARX模式相較於CHIM模式,精確度較高,且更能掌握淹水變化趨勢,故非常適合用於淹水預測,但所需儲存之模式參數量卻遠多於CHIM,對於模式資料庫負荷較大。 In recent years, the increasing frequency and severity of floods caused by climate change and/or land overuse has been reported both nationally and globally. Therefore, estimation of flood depths and extents may provide disaster information for alleviating risk and loss of life and property. The conventional inundation models need a huge amount of computational time to carry out the high-resolution spatial inundation maps. Moreover, for implementing appropriate mitigation strategies of various flood conditions, different flood scenarios and the corresponding mitigation alternatives are required. Consequently, it is very difficult to reach real-time simulation and/or forecast of the inundation extent by using conventional inundation models. In order to build real-time flood forecast systems, this study proposed two models, CHIM and SOM-R-NARX, for forecasting regional flood inundation depths and extents. The CHIM (clustering-based hybrid inundation model) model has two-stage procedure, including data preprocessing and model building stages. In the data preprocessing stage, K-means clustering is used to categorize the data points of the different flooding characteristics in the study area and to identify the control point(s) from individual flooding cluster(s). In the model building stage, R-NARX (recurrent configuration of nonlinear autoregressive with exogenous inputs) flood depth forecasting models are built for control point(s) and regional grids in each cluster(s). The SOM-R-NARX model is composed of SOM (Self-Organizing Map) and R-NARX. The SOM network categorizes different flood inundation maps of the study area to produce a regional flood topological map. The R-NARX model is built to forecast total inundated volume of the study area. To find the neuron with the closest total inundated volume to the forecasted total inundated volumes, the forecasted value is used to adjust the weights (inundated depths) of the closest neuron and obtain a regional flood inundation map. The major difference between these two models is that CHIM classify flooding characteristics, and SOM-R-NARX investigate the relationship between rainfall pattern and flooding spatial distribution. The practicability and accuracy of the proposed methodology is evaluated in Yilan county. The results show that the two proposed models can provide 3-h-ahead flood inundation maps efficiently and adequately. For comparison, SOM-R-NARX consistently outperform CHIM and can be adequately applied to online multistep-ahead forecasts of inundation depths in the study area. However, SOM-R-NARX needs more storage for model parameter than CHIM, and increases the loading of database as well. |
Appears in Collections: | [水資源及環境工程學系暨研究所] 學位論文
|
Files in This Item:
File |
Size | Format | |
index.html | 0Kb | HTML | 222 | View/Open |
|
All items in 機構典藏 are protected by copyright, with all rights reserved.
|