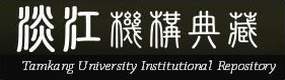 |
English
|
正體中文
|
简体中文
|
Items with full text/Total items : 62830/95882 (66%)
Visitors : 4152141
Online Users : 716
|
|
|
Loading...
|
Please use this identifier to cite or link to this item:
https://tkuir.lib.tku.edu.tw/dspace/handle/987654321/125168
|
Title: | RLR: Joint Reinforcement Learning and Attraction Reward for Mobile Charger in Wireless Rechargeable Sensor Networks |
Authors: | Shang, C.;Chang, C. Y.;Liao, W.-H.;Roy, D. S. |
Keywords: | Mobile charger;recharging mechanism;reinforcement learning;wireless rechargeable sensor network (WRSN) |
Date: | 2023-09-15 |
Issue Date: | 2024-03-07 12:05:56 (UTC+8) |
Publisher: | Institute of Electrical and Electronics Engineers |
Abstract: | Advances in wireless charging technology give great new opportunities for extending the lifetime of a wireless sensor network (WSN) which is an important infrastructure of IoT. However, the existing greedy algorithms lacked learning from the experiences of energy dissipation trends. Unlike the existing studies, this article proposes a reinforcement learning approach, called reinforcement learning recharging (RLR), for mobile charger to learn the trends of WSNs, including the energy consumption of the sensors, the recharging cost as well as the coverage benefit, aiming to maximize the coverage contribution of the recharged WSN. The proposed RLR mainly consists of three modules, including sensor energy management (SEM), charger location update (CLP), and charger reinforcement learning (CRL) modules. In the SEM module, each sensor manages its energy and calculates its threshold for the recharging request in a distributed manner. The CLP module adopts the quorum system to ensure effective communication between sensors and the mobile charger. Meanwhile, the CRL module employs attraction rewards to reflect the coverage benefit and penalties of waiting time raised due to charger movement and recharging other sensors. As a result, the charger accumulates the learning experiences from the Q -Table such that it is able to execute the appropriate actions of charging or moving in a manner of state management. Performance results show that the proposed RLR outperforms the existing recharging mechanisms in terms of charging waiting time of sensors, the energy usage efficiency of the mobile charger, as well as the coverage contribution of the given sensor network. |
Relation: | IEEE Internet of Things Journal 10(18), p. 16107-16120 |
DOI: | 10.1109/JIOT.2023.3267242 |
Appears in Collections: | [Graduate Institute & Department of Computer Science and Information Engineering] Journal Article
|
Files in This Item:
File |
Description |
Size | Format | |
index.html | | 0Kb | HTML | 3 | View/Open |
|
All items in 機構典藏 are protected by copyright, with all rights reserved.
|