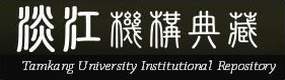 |
English
|
正體中文
|
简体中文
|
Items with full text/Total items : 64191/96979 (66%)
Visitors : 8222462
Online Users : 7410
|
|
|
Loading...
|
Please use this identifier to cite or link to this item:
https://tkuir.lib.tku.edu.tw/dspace/handle/987654321/124321
|
Title: | Real-time image-based air quality estimation by deep learning neural networks |
Authors: | Kow, Pu-Yun;Hsia, I-Wen;Chang, Li-Chiu;Chang, Fi-John |
Date: | 2022-01-24 |
Issue Date: | 2023-08-01 12:05:14 (UTC+8) |
Abstract: | Air quality profoundly impacts public health and environmental equity. Efficient and inexpensive air quality monitoring instruments could be greatly beneficial for human health and air pollution control. This study proposes an image-based deep learning model (CNN−RC) that integrates a convolutional neural network (CNN) and a regression classifier (RC) to estimate air quality at areas of interest through feature extraction from photos and feature classification into air quality levels. The models were trained and tested on datasets with different combinations of the current image, the baseline image, and HSV (hue, saturation, value) statistics for increasing model reliability and estimation accuracy. A total of 3549 hourly air quality datasets (including photos, PM2.5, PM10, and the air quality index (AQI)) collected at the Linyuan air quality monitoring station of Kaohsiung City in Taiwan constituted the case study. The main breakthrough of this study is to timely produce an accurate image-based estimation of several pollutants simultaneously by using only one single deep learning model. The test results show that estimation accuracy in terms of R2 for PM2.5, PM10, and AQI based on daytime (nighttime) images reaches 76% (83%), 84% (84%), and 76% (74%), respectively, which demonstrates the great capability of our method. The proposed model offers a promising solution for rapid and reliable multi-pollutant estimation and classification based solely on captured images. This readily scalable measurement approach could address major gaps between air quality data acquired from expensive instruments worldwide. |
Relation: | Journal of Environmental Management 307, 114560 |
DOI: | 10.1016/j.jenvman.2022.114560 |
Appears in Collections: | [人工智慧學系] 期刊論文
|
Files in This Item:
File |
Description |
Size | Format | |
index.html | | 0Kb | HTML | 121 | View/Open |
|
All items in 機構典藏 are protected by copyright, with all rights reserved.
|