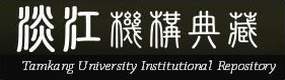 |
English
|
正體中文
|
简体中文
|
Items with full text/Total items : 62830/95882 (66%)
Visitors : 4107318
Online Users : 802
|
|
|
Loading...
|
Please use this identifier to cite or link to this item:
https://tkuir.lib.tku.edu.tw/dspace/handle/987654321/121525
|
Title: | Data Augmentation for a Deep Learning Framework for Ventricular Septal Defect Ultrasound Image Classification |
Authors: | Chen, Shih-Hsin;Tai, I-Hsin;Chen, Yi-Hui;Weng, Ken-Pen;Hsieh, Kai-Sheng |
Keywords: | Ventricular septal defect (VSD);Echo;Deep learning;Classification;Data augmentation |
Date: | 2021-01-10 |
Issue Date: | 2021-10-20 12:10:21 (UTC+8) |
Abstract: | Congenital heart diseases (CHD) can be detected through ultrasound imaging. Although ultrasound can be used for immediate diagnosis, doctors require considerable time to read dynamic clips; typically, physicians must continuously examine disease data from beating heart images. Most importantly, this type of diagnosis relies heavily on the expertise and experience of the diagnosing physician. This study established an ultrasound image classification with deep learning algorithms to overcome the challenges involved in CHD diagnosis. We detected the most common CHD, namely the first, second, and fourth types of ventricular septal defect (VSD). We improved the performance levels of well-known deep learning algorithms (InceptionV3, ResNet, and DenseNet). Because algorithm optimization and overfitting problems can influence the performance of deep learning algorithms, we studied some optimizer algorithms and early-stopping strategies. To enhance the solution quality, we used data augmentation methods for solving this classification problem. The selected approach was further compared with Google AutoML, which applies structure search for quality prediction. Our results revealed that the proposed deep learning algorithm was able to recognize most types of VSD. However, one type of VSD remains unconquered and warrants more advanced techniques. |
Relation: | Pattern Recognition. ICPR International Workshops and Challenges, p.310-322 |
DOI: | 10.1007/978-3-030-68799-1_22 |
Appears in Collections: | [資訊工程學系暨研究所] 會議論文
|
Files in This Item:
File |
Description |
Size | Format | |
index.html | | 0Kb | HTML | 44 | View/Open |
|
All items in 機構典藏 are protected by copyright, with all rights reserved.
|